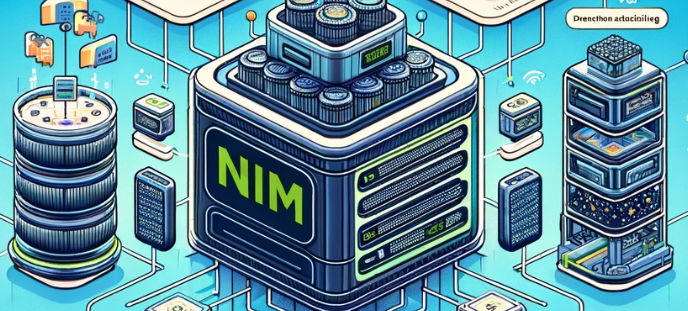
Making use of AI, and specifically Generative AI is being hailed as the ‘next informational revolution’, and for a whole range of industries being able to ask questions of data that you couldn’t ask yesterday is going to be hugely impactful.
However, in case you have not worked it out yet, it is very unlikely that organisations and enterprises are going to be building their own foundational models to drive those innovations. Two really obvious barriers stand in most organisations way, Complexity and Resources.
COMPLEXITY – These are incredibly complex models, building an AI foundation model involves navigating data acquisition and curation, model design, algorithm development – not to mention the ethical challenges of bias mitigation to ensure the model is representative and effective.
I was lucky enough to take a course run by the University of California on Computational Social Science delivered by Martin Hilbert which in particular explored the challenges of bias, representation and ethics – building you own model and being able to mitigate these challenges is non-trivial !
RESOURCES – The compute and power requirements for AI foundation models are significant, requiring extensive resources and energy for training and inference, which come at a high cost, environmental impact, and challenges in scaling.
Some of the figures involved in building out the platforms needed to train these models is mind-bending. Think 10,000 V100 GPUs running for six months and consuming 7000MWh, estimates put the cost of ChatGPT4 training cycles at around $100m – way out of reach of the average enterprise
… and even if you can resolve these issues, if you’re going to bring foundational models into your own world (vs train your own) how do you bridge the gap between the hugely complex world of AI development and the operational requirements of operations in the Enterprise IT world ?
The challenge is how to deal with the gap between delivering developer velocity for your new AI enabled workloads and apps, whilst operating within the constraints of your IT organisation.
Bridging that gap – NVIDIA NIM
On the opening Monday of GTC 2024 NVIDIA announced ‘NIM’, a new way to streamline custom and pre-trained AI models into production, and … proving that nothing in IT is ever new, the NIM is an optimised container image containing a pre-packaged model and inferencing engine – an “AI microservice” if you like.
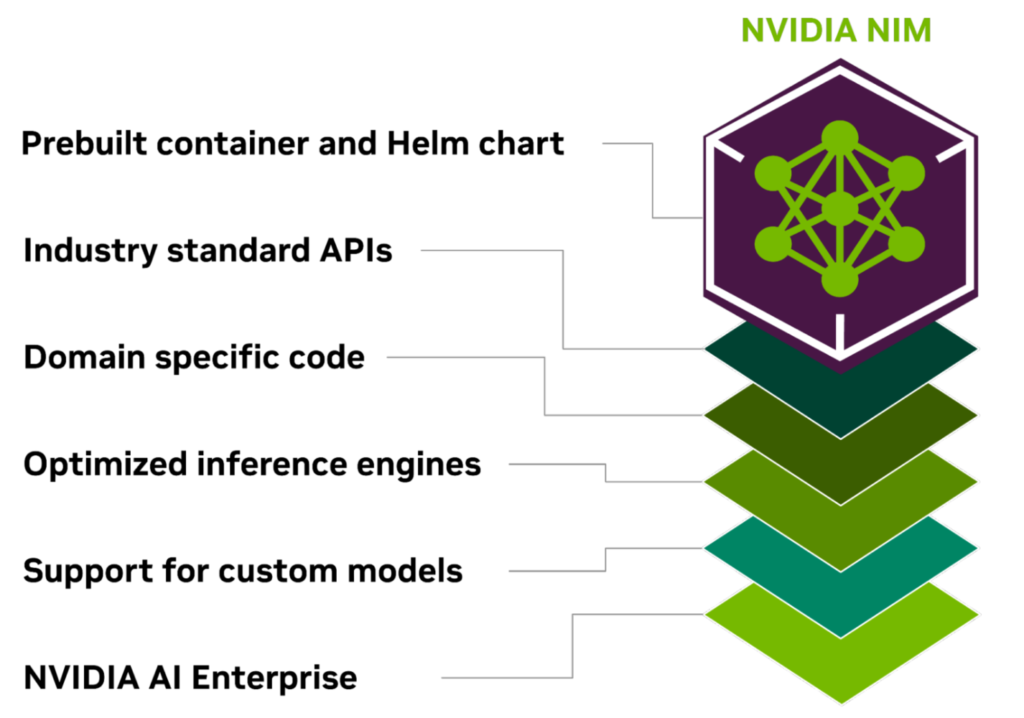
Portable, Packaged Simplicity
The result is a simple, packaged, portable container able to be run on local workstations, to Public Clouds and NVIDIA’s own systems – not to mention a whole range of vendor-developed platforms such as VMware’s Private AI Platform or Dell AI Factory with NVIDIA – both enabling enterprises to run RAG (Retrieval Augmented Generation) workflows, fine-tune and customize their models, and run inference workloads in their data centers where so often it is the location of the data (aka Data Gravity) that dictates where workloads are run – also allowing you to address the traditional Enterprise IT challenges of privacy, cost, performance, and compliance concerns. This is one of the reasons that we’re seeing companies like NetApp taking its pedigree in delivering cloud-led, data-centric solutions and enabling all that stored data to be funnelled into your AI strategy be that in the cloud, on prem or at the edge.
NVIDIA has developed NIMs with a number of Foundational Models across a range of modes including graphics and NVIDIA have already announced partnerships with the likes of A121, Cohere, Getty Images & Shutterstock alongside the open models that are available from Google, Hugging Face, Meta, Microsoft, Mistral AI and Stability AI.
Ultimately what NVIDIA is trying to do here is accelerate the rate at which Enterprises can adopt AI (and one might cynically say therefore aquire and purchase NVIDIA’s technology). This is about providing a helping hand to businesses looking to try to build an optimised efficient AI Infrastructure, cost effectively and get it into the hands of developers looking to use LLM and VLMs for developers whilst ensuring maximum portability and in a tightly opinionated approach.
Something to think about in closing though, is that for all the technology that’s in play here at the moment, doing this effectively and with impact is all about understanding your use case, and the use case of your customer. Only then can you work back, understand and identify the data, the skills, the teams (and the models) that you’ll need to pull together to achieve those goals.
Opinionated stacks like NIM from NVIDIA are a great way to remove a whole bunch of the variable that you can’t control and allow you to focus on your outcomes, the success metrics for those outcomes and execute a plan to achieve them without getting lost in the weeds.